Learning Strategy Fusion
Learning Strategy (LS) Fusion a method to fuse learning strategies (LSs) in reinforcement learning framework.
Generally, we need to choose a suitable LS for each task respectively.
In contrast, the proposed method automates this selection by fusing LSs.
The LSs fused in this research includes a transfer learning, a hierarchical RL, and a model based RL.
The proposed method has a wide applicability.
When the method is applied to a motion learning task, such as a crawling task, the performance of motion may be improved compared to an agent with a single LS.
The method also can be applied to a navigation task by hierarchically combining already learned motions, such as a crawling and a turning.
Actually, LS fusion was applied to a maze task of a humanoid robot where the robot learns not only a path to goal, but also a crawling and a turning motions.
Learning Strategy Fusion for Multiple Environment
We extended the Learning Strategy Fusion to learn policies across multiple types of environments.
The robot quickly adapts to a new environment with preserving policies of past environments. The proposed methods are verified with both a dynamics simulator and real robots.
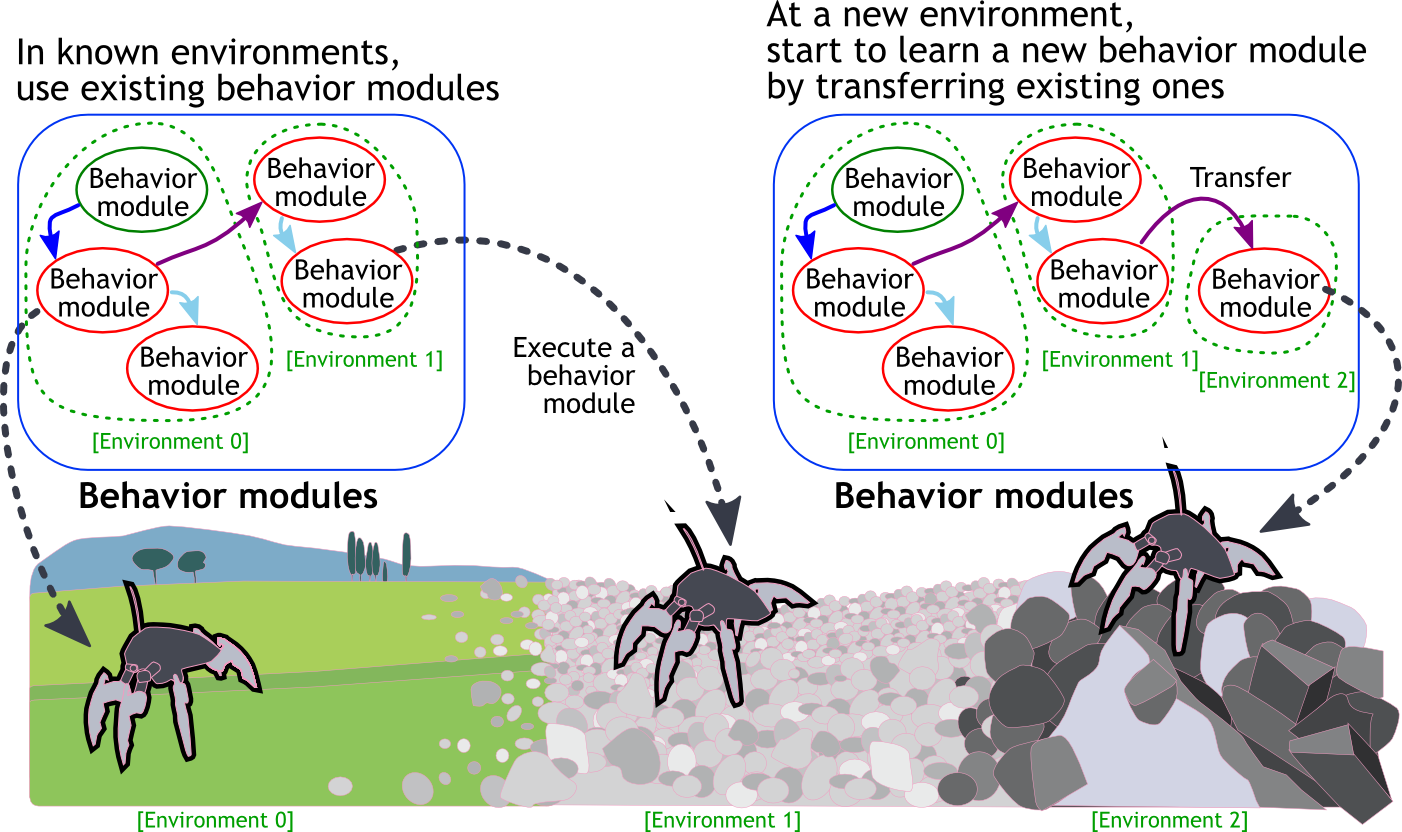
Fig: Conceptual diagram of the learning strategy fusion (left), and how it works in varying environments (right).
Crawling acquisition through 3 different terrains (learned from scratch):
Related Papers
- Akihiko Yamaguchi, Masahiro Oshita, Jun Takamatsu, and Tsukasa Ogasawara:
Experimental Verification of Learning Strategy Fusion for Varying Environments,
in Proceedings of the 10th ACM/IEEE International Conference on Human-Robot Interaction Extended Abstracts (HRI2015), pp. 171-172, Portland, 2015.
[final-draft]
[ACM]
- Akihiko Yamaguchi, Jun Takamatsu, and Tsukasa Ogasawara:
Learning Strategy Fusion to Acquire Dynamic Motion,
in Proceedings of the 11th IEEE-RAS International Conference on Humanoid Robots (Humanoids2011), pp.247-254, Bled, Slovenia, 2011.
[final-draft]
- Akihiko Yamaguchi, Jun Takamatsu, and Tsukasa Ogasawara:
Utilizing Dynamics and Reward Models in Learning Strategy Fusion,
in Proceedings of the 2011 JSME Conference on Robotics and Mechatronics (ROBOMEC2011), 1A1-O03, Okayama, Japan, May, 2011.
[PDF]
- Akihiko Yamaguchi, Jun Takamatsu, and Tsukasa Ogasawara:
Fusing Learning Strategies to Learn Various Tasks with Single Configuration,
IEICE Technical Report, Vol.110, No.461, NC2010-154, pp.159-164, March 2011. (in English)
[final-draft]
last updated at Sep. 18, 2013.